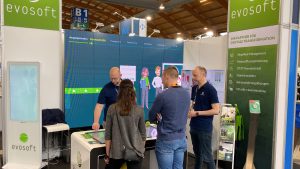
evosoft at “all about automation 2024” trade fair in Friedrichshafen
The “all about automation 2024” trade fair for industrial automation will take place in Friedrichshafen on March 5 and 6, 2024. evosoft will be represented as
The Industrial Internet of Things (IIoT) brings with it a multitude of new technical possibilities. The digital twin plays a decisive role in plant construction and mechanical engineering. It enables the virtual representation of physical objects, revolutionizes the life cycle of machines and systems and paves the way for a more efficient, data-driven future. How does a digital twin in the context of the Internet of Things change traditional approaches in plant and mechanical engineering and what concrete effects does this have on efficiency, innovation and the entire life cycle of industrial processes? How can you benefit from the potential applications and sustainably optimize your production?
The Internet of Things (IoT) is pushing the boundaries of technological innovation. The merging of the real and virtual worlds through the digital twin is fundamentally changing the way we design, develop and operate systems and machines. It also paves the way for a data-driven and therefore more efficient and precise future.
The term digital twin refers to the virtual representation of physical objects or systems, be it a machine, a plant or a product. In the context of mechanical and plant engineering, the digital twin enables an almost realistic simulation and analysis of all aspects throughout the entire life cycle of a unit. From virtual commissioning and the simulation of an entire factory or production process to predictive maintenance – the digital twin is revolutionizing this industry in many ways.
Today’s production facilities are no longer limited to the physical world. Assembly line robots, milling machines and automated warehouses actively send signals to local computers and servers, providing a continuous snapshot of the current status of a factory. This creates a continuous and open optimization cycle for both product and production. What opportunities does this create for your company?
The digital twin is having a revolutionary impact on plant construction and mechanical engineering in the IIoT by leading to an advanced transformation in various aspects. This includes virtual commissioning, factory simulation, monitoring and predictive maintenance as well as data-driven decision-making.
The integration of the digital twin enables the virtual commissioning of systems and machines even before their physical implementation. This innovative approach not only offers the opportunity to minimize risks, but also enables a comprehensive test phase, which in turn leads to accelerated and smooth physical commissioning.
By using virtual commissioning in the early phases of a development process, processes and automation can be validated. This makes it possible to identify potential errors at an early stage and make appropriate optimizations. Virtual simulation and validation therefore not only help to reduce costs, but also significantly shorten commissioning times. This preventative approach contributes to a more efficient and cost-effective realization of systems and machines.
Through integration into a specific IT landscape, factory simulation enables precise simulation and planning of production facilities, machines and processes based on individual requirements. By integrating real-time data from the real production environment into a simulation model, the digital twin provides valuable insights for strategic and operational business decisions. Virtual testing also enables a precise prediction of the future performance of the production plant and allows continuous optimization during operation.
Using this approach makes it possible to answer questions such as “When will a possible return on investment (ROI) be achieved with a partial modernization?” or “Does the plant create the necessary and desired output?” without having to carry out costly real implementations. This enables you to increase your efficiency, identify production bottlenecks and develop optimal production processes.
Performance forecasts with the Digital twin
Performance forecasts with the Digital twin
When is a possible return on investment (ROI) achieved with a partial modernization?
How can production be optimized?
Does the system provide the required / desired output?
Which measure is most effective for the production process?
How can the system be put into operation faster / more efficiently?
Why is it that production is not delivering the expected performance?
Does the system provide the required / desired output?
Does the system provide the required / desired output?
Does the system provide the required / desired output?
Does the system provide the required / desired output?
Furthermore, a digital twin opens up the possibility of continuous real-time monitoring of systems and machines. This makes it possible to record and analyze a wealth of data in real time. This continuous analysis allows potential problems and deviations from normal operating conditions to be identified at an early stage. This proactive approach makes it possible to anticipate emerging difficulties before serious malfunctions or breakdowns occur.
The early detection of potential problems by the digital twin leads to predictive maintenance. By integrating analysis results into a preventive maintenance approach, necessary maintenance measures can be planned in advance. This in turn significantly reduces unplanned downtimes and helps to extend the service life of systems and machines. Overall, the digital twin not only enables the optimization of operating processes, but also a significant increase in the reliability and efficiency of industrial plants in plant and mechanical engineering.
Optimize your system performance and reduce your operating costs at the same time with a digital twin! Find out in our webinar how this enables continuous optimization and troubleshooting throughout the entire product life cycle. Future-proof your company – get access now!
The combination of machine learning and the digital twin has the potential to bring about a comprehensive transformation in various industries. In virtual commissioning, machine learning in the digital twin could lead to improved simulation by helping to create more realistic scenarios and map dynamic processes more accurately. Through continuous learning, the digital twin can generate even more precise predictions for the factory simulation over time, which can lead to a highly realistic representation of the production environment.
The integration of machine learning into monitoring and predictive maintenance could open up new horizons for proactive maintenance. Through continuous data analysis, the digital twin can recognize patterns that indicate potential failures or maintenance needs. This enables predictive maintenance, where repairs can be planned in advance to minimize downtime and maximize the service life of equipment.
When it comes to optimizing processes and resources, machine learning in the digital twin can help to identify and continuously exploit efficiency reserves. By analysing large volumes of data, machine learning algorithms can understand complex correlations and uncover optimization potential for production processes. This enables dynamic adaptation of operating processes in order to use resources even more efficiently and optimize production targets.
For data-supported decision-making, the integration of machine learning means that the digital twin is not only able to provide precise information, but is also able to learn continuously. Machine learning algorithms can analyze complex correlations in real time and provide valuable insights for strategic and operational decisions. This enables more agile and data-based corporate management, as decisions are based on up-to-date, precise information.
Overall, the integration of machine learning into the digital twin in plant and mechanical engineering could create an intelligent, adaptive and self-learning environment. This development promises not only an increase in efficiency and productivity, but also increased flexibility and adaptability in a constantly changing production environment of digital transformation.
Simulation of production scenarios: Optimization of a machine park for wire harness production
The simulation model developed has created a clear target image for the reorganization of the machinery in wire harness production, on the basis of which different production orders can be realistically…
Suggested contributions
evosoft at “all about automation 2024” trade fair in Friedrichshafen
The “all about automation 2024” trade fair for industrial automation will take place in Friedrichshafen on March 5 and 6, 2024. evosoft will be represented as
evosoft technical lecture at “all about automation 2024” in Friedrichshafen
From March 05 – 06, 2024, the all about automation 2024 will take place in Friedrichshafen. There you will have the opportunity to attend the technical
evosoft is participant in the Alliance for Cyber Security
evosoft has become a member of the Alliance for Cyber Security. The Alliance is an initiative of the German Federal Office for Information Security (BSI), which